Expert Choice V11 Exercises
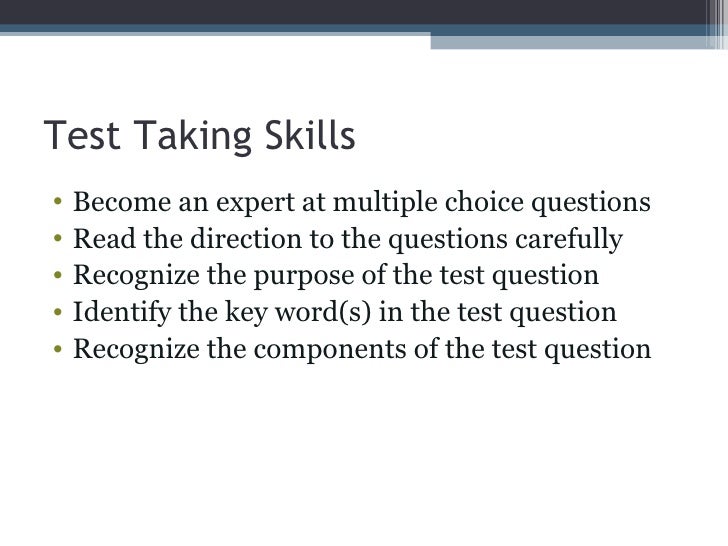
Portable Expert Choice 11 Full Version Merupakan Software Portable, Tidak Perlu Install, Langsung Dipakai Lebih Praktis, Bisa Disimpan Di Flaskdisk, Komputer, Laptop, DLL. The Kirkland Signature Choice app is compatible with Kirkland Signature 6.0 hearing aids.The Kirkland Signature Choice app lets you control your h. Free Publisher: GN Hearing Downloads: 2.
Expert Choice V11 Exercises Free
Note Response Surface Methods: If you wish to experiment on a continuous factor, such as time, which can be adjusted to any numerical level, consider using response surface methods (RSM) instead. This is covered in a. The data for this example come from the Stat-Ease bowling league. Three bowlers (Pat, Mark, and Shari) are competing for the last team position. They each bowl six games in random order — ideal for proper experimentation protocol.
Results are: Game Pat Mark Shari 1 160 165 166 2 150 180 158 3 140 170 145 4 167 185 161 5 157 195 151 6 148 175 156 Mean 153.7 178.3 156.2 Bowling scores Being a good experimenter, the team captain knows better than to simply pick the bowler with the highest mean score. The captain needs to know if the average scores are significantly different, given the variability in individual games. Maybe it’s a fluke that Mark’s score is highest. This one-factor case study provides a good introduction to the power of simple comparative design of experiments (DOE). It exercises many handy features found in Design-Expert software. Response name dialog box - completed At this stage you can skip the remainder of the fields and continue on. However, it is good to gain an assessment of the power of your planned experiment.
In this case, as shown in the fields below, enter the value 20 for the signal because the bowling captain does not care if averages differ by fewer than 20 pins. Then enter the value 10 for standard deviation (derived from league records as the variability of a typical bowler).
Design-Expert then computes a signal-to-noise ratio of 2 (20 divided by 10). Enter the Response Data When performing your own experiments, you will need to go out and collect the data. Simulate this by exiting out of the program. Click on Yes if you are prompted to Save. Now re-start Design-Expert and click on Open Design (or click the open file icon on the toolbar) to open the data file you saved before ( Bowling.dxpx). You should now see your data tabulated in the randomized layout. For this example, you must enter your data in the proper order to match the correct bowlers.
To do this, right-click the Factor 1 (A: Bowler) column header and choose Sort Ascending. Note Advantages of being accurate on the actual run order: If you are a real stickler, replace (type over) your run numbers with the ones shown above, thus preserving the actual bowlers’ game sequence. Bowling six games is taxing but manageable for any serious bowler.
However, short and random breaks while bowling six games protects against time-related effects such as learning curve (getting better as you go) and/or fatigue (tiring over time). Save your data by selecting File, Save from the menu (or via the save icon on the toolbar). Now you’re backed up in case you mess up your data. This backup is good because now we’ll demonstrate many beneficial procedures Design-Expert features in its design layout.
For example, right-click the top left cell of the table. This allows you to control what Design-Expert displays. For this exercise, choose Comments. Note ANOVA annotation: Now select View, Show Annotation from the menu atop the screen and uncheck this option. Note that the textual hints and explanations disappear so you can make a clean printout for statistically savvy clients. Re-select View, Show Annotation to ‘toggle’ back all the helpful hints.
Before moving on, try right-clicking on the p-value of 0.0006 as shown above (select Help at the bottom of the pop-up menu). There’s a wealth of information to be brought up from within the program with a few simple clicks: Take advantage! Now, look to the right side of your screen at the Fit Statistics pane to see various summary statistics. Coefficient estimates Here you see statistical details such as coefficient estimates for each model term and their confidence intervals (“CI”).
The intercept in this simple one-factor comparative experiment is just the overall mean score of the three bowlers. You may wonder why only two terms, A1 and A2, are provided for a predictive model on three bowlers. It turns out that the last model term, A3, is superfluous because it can be inferred once you know the mean plus the averages of the other two bowlers. Now let’s move on to the next section within this screen: “Treatment Means.” Click the Treatment Means tab in the ANOVA pane.
Note The ‘pencil test’: If you have a pencil handy (or anything straight), hold it up to the graph. Does it loosely cover up all the points? The answer is “Yes” in this example – it passes the “pencil test” for normality. You can reposition the thin red line by dragging it or its “pivot point” (the round circle in the middle). However, we don’t recommend you bother doing this – the program generally places the line in the ideal location automatically.
If you need to reset the line, simply double-click your left mouse button over the graph. Notice that the points are coded by color to the level of response they represent – going from cool blue for lowest values to hot red for the highest.
In this example, the red point is Mark’s outstanding 195 game. Pat and Shari think Mark’s 195 game should be thrown out because it’s too high. Is this fair? Click this point so it will be selected on this and all the other residual graphs on the Diagnostics Tool (choose how many graphs are displayed at once via the blue layout icons above the Diagnostics tab). Other ways to display residuals In any case, when runs have greater leverage (another statistical term to look up in the Help), only the Studentized form of residuals produces valid diagnostic graphs.
For example, if Pat and Shari succeed in getting Mark’s high game thrown out (don’t worry – they won’t!), then each of Mark’s remaining five games will exhibit a leverage of 0.2 (1/5) versus 0.167 (1/6) for each of the others’ six games. Due to potential imbalances of this sort, we advise that you always leave the Studentized feature checked (as done by default). So if you are on Residuals now, go back to the original choice that came up by default (externally. studentized).
Another aspect of how Design-Expert displays residuals by default is them being done “externally”. This is explored in the For now, suffice it to say that the program chooses this form of residual to provide greater sensitivity to statistical outliers. This makes it even more compelling not to throw out Mark’s high game.
Now select the Resid. Tab to view a plot of residuals for each individual game versus what is predicted by the response model.

Residuals versus predicted values, colored by bowler The size of the studentized residual should be independent of its predicted value. In other words, the vertical spread of the studentized residuals should be approximately the same for each bowler. In this case the plot looks OK. Don’t be alarmed that Mark’s games stand out as a whole. The spread from bottom-to-top is not out of line with his competitors, despite their protestations about the highest score (still highlighted). Bring up the next graph on the list – Resid.
Vs Run (residuals versus run number). Note Repercussion of possible trends: In this example, things look relatively normal. However, even if you see a pronounced upward, downward, or shift change, it will probably not bias the outcome because the runs are completely randomized. To ensure against your experiment being sabotaged by uncontrolled variables, always randomize! More importantly in this case, all points fall within the limits (calculated at the 95 percent confidence level).
In other words, Mark’s high game does not exhibit anything more than common-cause variability, so it should not be disqualified. Note Individual comparisons on the model graph: If you click on one of the boxes at the center of the LSD bars representing the mean, pairwise comparisons will be graphically displayed.
A horizontal line is drawn through the predicted mean of the highlighted point. Any vertical bars that overlap with this horizontal line indicate predicted means that are not significantly different from the selected point. The legend will also tabulate which means are significantly different. Note that even though the displayed pairwise tests are two-sided, only half of the interval is displayed for easier interpretation. Pat and Shari’s LSD bars overlap horizontally, so we can’t say which of them bowls better. It seems they must spend a year in a minor bowling league and see if a year’s worth of games reveals a significant difference in ability.
Meanwhile, Mark will be trying to live up to the high average he exhibited in the tryouts and thus justify being chosen for the Stat-Ease bowling team. That’s it for now.
Save your results by going to File, Save (or by clicking the icon). You can now Exit Design-Expert if you like, or keep it open and go on to the next tutorial – part two for general one-factor design and analysis. It delves into advanced features via further adventures in bowling.
Note Due to the specific nature of this case study, a number of features that could be helpful to you for RSM will not be implemented in this tutorial. Many of these features are used in the earlier tutorials. If you have not completed all these tutorials, consider doing so before starting this one. We will presume that you are knowledgeable of the statistical aspects of RSM. For a good primer on the subject, see RSM Simplified (Anderson and Whitcomb, Productivity, Inc., New York, 2005). You will find overviews on RSM and how it’s done via Design-Expert in the on-line Help system.
To gain a working knowledge of RSM, we recommend you attend our Response Surface Methods for Process Optimization workshop. Call Stat-Ease or visit our website for a schedule at www.statease.com. The case study in this tutorial involves production of a chemical. The two most important responses, designated by the letter “y”, are.
y1 - Conversion (% of reactants converted to product). y2 - Activity. The experimenter chose three process factors to study. Their names and levels are shown in the following table. Factor Units Low Level (-1) High Level (+1) A - Time minutes 40 50 B - Temperature degrees C 80 90 C - Catalyst percent 2 3 Factors for response surface study You will study the chemical process using a standard RSM design called a central composite design (CCD). It’s well suited for fitting a quadratic surface, which usually works well for process optimization. Default CCD option for alpha set so design is rotatable Many options are statistical in nature, but one that produces less extreme factor ranges is the “Practical” value for alpha.
This is computed by taking the fourth root of the number of factors (in this case 3¼ or 1.31607). See RSM Simplified Chapter 8 “Everything You Should Know About CCDs (but dare not ask!)” for details on this practical versus other levels suggested for alpha in CCDs – the most popular of which may be the “Face Centered” (alpha equals one). Press OK to accept the rotatable value. (Note: you won’t get the “center points in each axial block” option until you change to 2 blocks in this design, as below). Using the information provided in the table on page 1 of this tutorial (or on the screen capture below), type in the details for factor Name (A, B, C), Units, and Low and High levels. Enter the Response Data – Create Simple Scatter Plots Assume that the experiment is now completed.
At this stage, the responses must be entered into Design-Expert. We see no benefit to making you type all the numbers, particularly with the potential confusion due to differences in randomized run orders.

Therefore, use the Help, Tutorial Data menu and select Chemical Conversion from the list. Let’s examine the data!
Click on the Design node on the left to view the design spreadsheet. Move your cursor to Std column header and right-click to bring up a menu from which to select Sort Ascending (this can also be done via a double-click on the header). Displaying the Point Type Notice the new column identifying points as “Factorial,” “Center” (for center point), and so on. Notice how the factorial points align only to the Day 1 block.
Download Expert Choice
Then in Day 2 the axial points are run. Center points are divided between the two blocks. Unless you change the default setting for the Select option, do not expect the Type column to appear the next time you run Design-Expert. It is only on temporarily at this stage for your information. Before focusing on modeling the response as a function of the factors varied in this RSM experiment, it will be good to assess the impact of the blocking via a simple scatter plot. Click the Graph Columns node branching from the design ‘root’ at the upper left of your screen.
You should see a scatter plot with factor A:Time on the X-axis and the Conversion response on the Y-axis. Note The correlation grid that pops up with the Graph Columns can be very interesting.
First off, observe that it exhibits red along the diagonal—indicating the complete (r=1) correlation of any variable with itself (Run vs Run, etc). Block versus run (or, conversely, run vs block) is also highly correlated due to this restriction in randomization (runs having to be done for day 1 before day 2). It is good to see so many white squares because these indicate little or no correlation between factors, thus they can be estimated independently. For now, it is most useful to produce a plot showing the impact of blocks because this will be literally blocked out in the analysis. Therefore, on the floating Graph Columns tool click the button where Conversion intersects with Block as shown below. Begin analysis of Conversion Design-Expert provides a full array of response transformations via the Transform option.
Click Tips for details. For now, accept the default transformation selection of None. Now click the Fit Summary tab. At this point Design-Expert fits linear, two-factor interaction (2FI), quadratic, and cubic polynomials to the response. At the top is the response identification, immediately followed below, in this case, by a warning: “The Cubic Model is aliased.” Do not be alarmed.
By design, the central composite matrix provides too few unique design points to determine all the terms in the cubic model. It’s set up only for the quadratic model (or some subset). Next you will see several extremely useful tables for model selection. Each table is discussed briefly via sidebars in this tutorial on RSM. Note The Sequential Model Sum of Squares table: The model hierarchy is described below:. “Linear vs Block”: the significance of adding the linear terms to the mean and blocks,.
“2FI vs Linear”: the significance of adding the two factor interaction terms to the mean, block, and linear terms already in the model,. “Quadratic vs 2FI”: the significance of adding the quadratic (squared) terms to the mean, block, linear, and twofactor interaction terms already in the model,.
“Cubic vs Quadratic”: the significance of the cubic terms beyond all other terms. Fit Summary tab For each source of terms (linear, etc.), examine the probability (“Prob F”) to see if it falls below 0.05 (or whatever statistical significance level you choose). So far, Design-Expert is indicating (via bold highlighting) the quadratic model looks best – these terms are significant, but adding the cubic order terms will not significantly improve the fit. (Even if they were significant, the cubic terms would be aliased, so they wouldn’t be useful for modeling purposes.) Move down to the Lack of Fit Tests pane for Lack of Fit tests on the various model orders. The “Lack of Fit Tests” pane compares residual error with “Pure Error” from replicated design points. If there is significant lack of fit, as shown by a low probability value (“ProbF”), then be careful about using the model as a response predictor. In this case, the linear model definitely can be ruled out, because its Prob F falls below 0.05.
The quadratic model, identified earlier as the likely model, does not show significant lack of fit. Remember that the cubic model is aliased, so it should not be chosen. Look over the last pane in the Fit Summary report, which provides “Model Summary Statistics” for the ‘bottom line’ on comparing the options The quadratic model comes out best: It exhibits low standard deviation (“Std. Dev.”), high “R-Squared” values, and a low “PRESS.” The program automatically underlines at least one “Suggested” model. Always confirm this suggestion by viewing these tables. The options for process order Also, you could now manually reduce the model by clicking off insignificant effects.
For example, you will see in a moment that several terms in this case are marginally significant at best. Design-Expert provides several automatic reduction algorithms as alternatives to the “Manual” method: “Backward,” “Forward,” and “Stepwise.” Click the “Auto Select” button to see these. From more details, try Screen Tips and/or search Help. Click the ANOVA tab to produce the analysis of variance for the selected model.
Statistics for selected model: ANOVA table The ANOVA in this case confirms the adequacy of the quadratic model (the Model ProbF is less than 0.05.) You can also see probability values for each individual term in the model. You may want to consider removing terms with probability values greater than 0.10. Use process knowledge to guide your decisions. Next, move over to the Fit Statistics pane to see that Design-Expert presents various statistics to augment the ANOVA. The R-Squared statistics are very good — near to 1. Cook’s Distance — the first of the Influence diagnostics Nothing stands out here.
Move on to the Leverage tab. This is best explained by the previous tutorial on One-Factor RSM so go back to that if you did not already go through it. Then skip ahead to DFBETAS, which breaks down the changes in the model to each coefficient, which statisticians symbolize with the Greek letter β, hence the acronym DFBETAS — the difference in betas.
For the Term click the down-list arrow and select A as shown in the following screen shot. Note Click outside the Term field, reposition your mouse over the Term field and simply scroll your mouse wheel to quickly move up and down the list. In a similar experiment to this one, where the chemist changed catalyst, the DFBETAS plot for that factor exhibited an outlier for the one run where its level went below a minimal level needed to initiate the reaction.
Thus, this diagnostic proved to be very helpful in seeing where things went wrong in the experiment. Now move on to the Report tab in the bottom-rite pane to bring up detailed case-by-case diagnostic statistics, many which have already been shown graphically. Note Design-Expert displays any actual point included in the design space shown. In this case you see a plot of conversion as a function of time and temperature at a mid-level slice of catalyst. This slice includes six center points as indicated by the dot at the middle of the contour plot. By replicating center points, you get a very good power of prediction at the middle of your experimental region.
The Factors Tool appears on the right with the default plot. Move this around as needed by clicking and dragging the top blue border (drag it back to the right side of the screen to “pin” it back in place. The tool controls which factor(s) are plotted on the graph. Note Each factor listed in the Factors Tool has either an axis label, indicating that it is currently shown on the graph, or a slider bar, which allows you to choose specific settings for the factors that are not currently plotted.
All slider bars default to midpoint levels of those factors not currently assigned to axes. You can change factor levels by dragging their slider bars or by left-clicking factor names to make them active (they become highlighted) and then typing desired levels into the numeric space near the bottom of the tool. Give this a try. Click the C: Catalyst toolbar to see its value. Don’t worry if the slider bar shifts a bit — we will instruct you how to re-set it in a moment. Note To enable a handy tool for reading coordinates off contour plots, go to View, Show Crosshairs Window (click and drag the titlebar if you’d like to unpin it from the left of your screen).
Intel play qx3 microscope manual. To download, select the best match for your device. To see more matches, use our custom search engine to. Or you can and we will find it for you. Discuss driver problems and ask for help from our community on our. Then click the Download button.
Now move your mouse over the contour plot and notice that Design-Expert generates the predicted response for specific factor values corresponding to that point. If you place the crosshair over an actual point, for example – the one at the far upper left corner of the graph now on screen, you also see that observed value (in this case: 66). Factors sheet In the columns labeled Axis and Value you can change the axes settings by right-clicking, or type in specific values for factors.
Give this a try. Then close the window and press the Default button. The Terms list on the Factors Tool is a drop-down menu from which you can also select the factors to plot. Only the terms that are in the model are included in this list.
At this point in the tutorial this should be set at AB. If you select a single factor (such as A) the graph changes to a One-Factor Plot. Try this if you like, but notice how Design-Expert warns if you plot a main effect that’s involved in an interaction.
The Perturbation plot with factor A clicked to highlight it For response surface designs, the perturbation plot shows how the response changes as each factor moves from the chosen reference point, with all other factors held constant at the reference value. Design-Expert sets the reference point default at the middle of the design space (the coded zero level of each factor). Click the curve for factor A to see it better. The software highlights it in a different color as shown above. It also highlights the legend.
(You can click that too – it is interactive!) In this case, at the center point, you see that factor A (time) produces a relatively small effect as it changes from the reference point. Therefore, because you can only plot contours for two factors at a time, it makes sense to choose B and C – and slice on A.